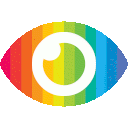
1. The factorization problem, which involves separating the causes of a raw sensory signal that contain multiple attributes, is at the core of perception and cognition.
2. An in-memory factorizer has been proposed that efficiently factorizes product vectors to obtain estimates of constituent attributes using a resonator network and memristive devices for in-memory computing.
3. The intrinsic stochasticity associated with memristive devices can substantially reduce limit cycles and enable exploration of a larger solution space, making the in-memory factorizer an effective tool for breaking down complex perceptual representations.
The article discusses a new approach to solving the factorization problem in sensory perception using high-dimensional holographic vectors and in-memory computing. The proposed non-deterministic, non-von Neumann compute engine combines an enhanced variant of a resonator network with sparse activations and memristive devices to efficiently factorize product vectors into their constituent attributes. The article provides a detailed explanation of the mathematical approach and the technical implementation of the proposed solution.
Overall, the article appears to be well-researched and informative, providing a thorough explanation of the proposed solution. However, there are some potential biases and limitations that should be considered.
One potential bias is that the article focuses primarily on the technical aspects of the proposed solution, without discussing its broader implications or potential limitations. For example, while the article notes that future artificial intelligence systems could benefit from this technology, it does not explore any potential risks or ethical considerations associated with such systems.
Additionally, while the article provides a detailed explanation of how holographic vectors can be used to represent combinations of attributes, it does not discuss any alternative approaches or potential drawbacks to this method. Similarly, while the article explains how memristive devices can be used for in-memory computing, it does not explore any potential limitations or challenges associated with this technology.
Overall, while the article provides valuable insights into a promising new approach to solving the factorization problem in sensory perception using holographic vectors and in-memory computing, readers should consider its potential biases and limitations when evaluating its claims.