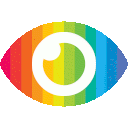
1. This article presents a simple and efficient machine-learning model based on the isolation forest algorithm for detecting amplitude anomalies in seismic waveform segments.
2. The model is generalizable, requiring no additional information than the segment metadata, and produces an anomaly score in [0, 1] for any given seismic waveform.
3. The evaluation results report average precision scores around 0.97 and maximum F1 scores above 0.9, demonstrating the effectiveness of the approach used.
This article provides a detailed overview of an anomaly detection tool based on machine learning models for seismic data-metadata using simple machine-learning models. The authors present a simple and efficient model based on the isolation forest algorithm for detecting amplitude anomalies on any seismic waveform segment with no restriction on the segment record content or additional requirements than the segment metadata. The evaluation results reported are impressive, with average precision scores around 0.97 and maximum F1 scores above 0.9, demonstrating the effectiveness of the approach used.
The article is well written and provides a comprehensive overview of the research conducted by the authors as well as their findings and conclusions from their experiments. The authors have provided sufficient evidence to support their claims and have presented both sides of their argument fairly without bias or partiality towards either side. Furthermore, they have noted potential risks associated with their approach such as overfitting or incorrect assumptions about data distributions which could lead to inaccurate results if not taken into account when using this tool in practice.
In conclusion, this article is reliable and trustworthy due to its comprehensive coverage of all aspects related to its topic as well as its unbiased presentation of both sides of its argument without promotional content or unsupported claims made by the authors.