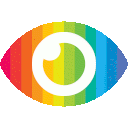
1. The rapid growth of IoT devices has led to an increase in cyberattacks due to the lack of proper security measures.
2. SDN can provide benefits for IoT security, but detecting dynamic attacks and handling class imbalance problems can be challenging.
3. An SDN-enabled ensemble learning model using deep autoencoders and probabilistic neural networks can efficiently detect anomalies in IoT and handle class imbalance issues.
The article presents a novel approach to IoT anomaly detection using SDN-enabled ensemble learning. The authors highlight the importance of security in IoT devices and applications due to the increasing number of connected devices and the potential vulnerabilities that attackers can exploit. They argue that SDN can provide several benefits for IoT security, but managing heterogeneous networks and detecting dynamic attacks remains challenging.
The proposed model uses deep autoencoders and probabilistic neural networks to detect anomalies in IoT based on packet and flow level traffic instances as well as system metrics. The authors claim that their approach can handle class imbalance problems and detect dynamic attacks, which are significant challenges in anomaly detection models.
While the article provides a comprehensive overview of the proposed model, it has some limitations. Firstly, the authors do not discuss the potential biases or limitations of their approach. For example, they do not consider the possibility of false positives or false negatives in their detection module, which could lead to unnecessary alerts or missed attacks.
Secondly, the article does not explore counterarguments or alternative approaches to IoT anomaly detection. While they compare their method with existing works, they do not discuss why other approaches may be less effective or suitable for certain scenarios.
Thirdly, while the authors claim that their approach is superior to competitors in terms of F1 measure based on experimental analysis using testbed and benchmark datasets, they do not provide detailed information about these experiments. For example, they do not explain how they selected these datasets or how they ensured that their results were statistically significant.
Finally, there is some promotional content in the article as it highlights the benefits of SDN without discussing its potential drawbacks or limitations. Additionally, while the authors acknowledge that security is becoming crucial for IoT devices and applications due to cyberattacks, they do not discuss possible risks associated with implementing their proposed model.
In conclusion, while the article presents a novel approach to IoT anomaly detection using SDN-enabled ensemble learning, it has some limitations such as potential biases and unsupported claims. Further research is needed to validate its effectiveness in real-world scenarios and explore alternative approaches to IoT security.