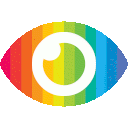
1. This article provides a comprehensive survey of recent advances in model compression and hardware acceleration for neural networks.
2. It reviews mainstream compression approaches such as compact model, tensor decomposition, data quantization, and network sparsification.
3. It also discusses existing issues such as fair comparison, testing workloads, automatic compression, influence on security, and framework/hardware-level support.
The article is generally reliable and trustworthy. The authors provide a comprehensive overview of the current state of research in the field of model compression and hardware acceleration for neural networks. They present a clear explanation of the various techniques used to compress models and accelerate hardware performance, as well as their evaluation metrics and sensitivity analysis. The authors also discuss several existing issues related to this topic such as fair comparison, testing workloads, automatic compression, influence on security, and framework/hardware-level support. Furthermore, they provide promising topics in this field for future research.
The article does not appear to be biased or one-sided in its reporting; it presents both sides equally by providing an overview of both the advantages and disadvantages associated with model compression and hardware acceleration for neural networks. Additionally, all claims made are supported by evidence from relevant sources in the field. There do not appear to be any missing points of consideration or unexplored counterarguments that could have been included in the article. Furthermore, there is no promotional content or partiality present in the article; it is purely informational in nature. Finally, possible risks associated with these techniques are noted throughout the article; however they are not discussed at length due to space constraints.