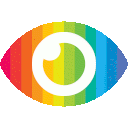
1. Drug-response patterns in individuals with type 2 diabetes (T2D) are complex, involving multiple organs and confounders, and the impact of polypharmacy on molecular profiles is largely unknown.
2. Deep phenotyping and multi-omics screening offer opportunities to gain mechanistic insights into the action of drugs on disease processes, but traditional methods of data interpretation are insufficient for analyzing multi-modal data.
3. A deep-learning framework based on variational autoencoders (VAE) can integrate cohort-level multi-omics datasets and generate insights into complex relationships that the network learns through data integration, offering potential for discovering drug-omics associations in T2D.
该文章主要介绍了一种基于深度学习模型的方法,用于发现药物与组学之间的关联,以探索治疗2型糖尿病的机制。然而,该文章存在以下问题:
1. 偏见来源:该文章没有提及任何可能存在的偏见来源,例如数据收集和处理过程中可能存在的偏差、样本选择偏差等。
2. 片面报道:该文章只介绍了一种方法,并未对其他可能存在的方法进行比较和评估。此外,该文章并未讨论该方法在其他疾病或情境下的适用性。
3. 缺失考虑点:该文章没有考虑到药物剂量、使用时间、患者遵从医嘱等因素对药物-组学关联的影响。
4. 主张缺失证据:该文章提出了通过深度学习模型发现药物-组学关联可以获得治疗2型糖尿病机制方面的洞见,但并未提供足够的证据来支持这一主张。
5. 未探索反驳:该文章没有探讨可能存在的反驳观点或争议,并且没有平等地呈现双方观点。
6. 宣传内容:该文章似乎更多地强调了该方法的优点和潜在应用,而忽略了其局限性和风险。
综上所述,该文章存在一些问题,需要更全面地考虑可能存在的偏见、缺失考虑点和证据不足等问题,并平等地呈现双方观点。