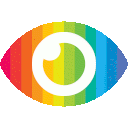
1. Meta AI has released two Llama 3 models, an 8 billion parameter model and a 70 billion parameter model, with a 405 billion parameter model on the horizon.
2. The 8 billion parameter model outperforms the largest Llama 2 models, indicating significant progress in AI capabilities.
3. The Llama 3 models are available in base and instruction-tuned formats, have a context length of 8K tokens, and have been trained on over 15 trillion tokens, nearly double the amount of previous models.
The article provides a detailed overview of Meta AI's release of the Llama 3 models, highlighting key features such as the 8 billion and 70 billion parameter models, training data, benchmarks, licensing conditions, and future prospects. While the article covers a wide range of information about the models, there are several areas where critical analysis is warranted.
One potential bias in the article is its focus on the positive aspects of the Llama 3 models without delving into potential drawbacks or limitations. The article emphasizes the models' performance in benchmarks and their competitive edge over other existing models but does not explore any potential weaknesses or areas where they may fall short. This one-sided reporting could give readers an overly optimistic view of the models without providing a balanced perspective.
Additionally, some claims made in the article lack sufficient evidence or context to support them. For example, when discussing the performance of the 8 billion parameter model compared to Mistral 7B and Gemma instruction-tuned models, specific data or metrics are not provided to back up these claims. Without concrete evidence to support these comparisons, readers may question the validity of these statements.
Furthermore, there are missing points of consideration in the article that could provide a more comprehensive understanding of the Llama 3 models. For instance, while the article mentions that the training data includes non-English tokens, it does not explore how this impacts the model's performance on multilingual tasks or whether there are any limitations in handling different languages. Additionally, there is no discussion on potential biases in the training data or how they may affect model outputs.
The article also lacks exploration of potential risks associated with using Llama 3 models. While it briefly mentions restrictions on improving other large language models using Llama 3's output, there is no discussion on ethical considerations or concerns related to AI bias, privacy issues, or misuse of AI technology. Providing a more thorough analysis of these risks would offer readers a more well-rounded view of the implications of using these advanced AI models.
Overall, while the article provides valuable information about Meta AI's Llama 3 models and their capabilities, there are areas where critical analysis is needed to address potential biases, unsupported claims, missing points of consideration, and unexplored counterarguments. By offering a more balanced perspective and addressing these gaps in information, readers can gain a deeper understanding of both the strengths and limitations of these cutting-edge AI models.