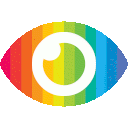
1. 本文介绍了可解释强化学习(XRL)的概念和分类,并提供了一些XRL模型的示例方法。
2. 解释性在人工智能中的重要性体现在用户信任、透明度和决策可证明等方面,也与法律规定和关键基础设施安全有关。
3. 文章强调了需要针对非专业用户进行适应的XAI/XRL模型,并对现有XRL方法进行了批判性评估。
The article "Explainable Reinforcement Learning: A Survey" provides a comprehensive overview of the need for explainable artificial intelligence (XAI) and its application in reinforcement learning (RL). However, the article lacks a critical analysis of potential biases and limitations in the presented information.
One potential bias is the focus on XAI models adapted to non-expert human end-users. While this is an important consideration, it may overlook the needs of expert users who require more complex and accurate models. Additionally, the article does not explore potential risks associated with XAI, such as unintended consequences or misuse by malicious actors.
The article also presents a one-sided view of the importance of transparency and explainability in AI systems. While transparency can increase trust and acceptance, it may not always be feasible or desirable in certain contexts, such as national security or trade secrets. The article could benefit from exploring these trade-offs more thoroughly.
Furthermore, some claims made in the article lack sufficient evidence or are presented without proper context. For example, the statement that "transparency has been identified as one key component...in increasing users' trust" is not supported by any specific studies or sources.
Overall, while "Explainable Reinforcement Learning: A Survey" provides valuable insights into XAI and RL, it would benefit from a more critical analysis of potential biases and limitations in its arguments.