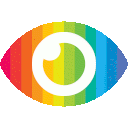
1. The competitiveness of listed companies in high-end equipment manufacturing industry was studied using random forest, a supervised machine learning algorithm based on regression and classification.
2. Enterprise scale accounts for a high proportion in the comprehensive competitiveness, and its score often affects the comprehensive strength; and the gap between companies in the same industry is also obvious.
3. The empirical evaluation results provide three enlightenments for enterprises to improve their comprehensive competitiveness, such as seizing the strategic opportunity to expand the market, expanding the scale of enterprises, improving asset management, and narrowing the industry gap.
The article titled "Prediction Algorithm of Collaborative Innovation Capability of High-End Equipment Manufacturing Enterprises Based on Random Forest" discusses the competitiveness of listed companies in the high-end equipment manufacturing industry using random forest. The paper provides insights into the comprehensive competitiveness among industries and how enterprise scale accounts for a high proportion in the comprehensive competitiveness.
The article provides a literature review that highlights the importance of technological innovation, collaboration between industry, university, and research institutions, and government policies to improve the level of technological innovation in the equipment manufacturing industry. However, there is no discussion on potential biases or limitations of these studies.
The methodology section explains the use of random forest for evaluating enterprise collaborative capabilities. The article claims that random forest can avoid overfitting and deal with high-dimensional data. However, there is no evidence provided to support these claims.
The article also discusses parameter optimization of the random forest model but does not provide any details on how this was done or what parameters were optimized.
Overall, while the article provides some useful insights into the competitiveness of listed companies in high-end equipment manufacturing industry using random forest, it lacks depth in its analysis and fails to address potential biases or limitations in its methodology. Additionally, there are unsupported claims made throughout the article without providing evidence to support them.