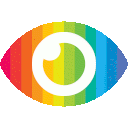
1. Language model pre-training has been shown to capture a surprising amount of world knowledge, which is necessary for NLP tasks such as question answering.
2. To make this knowledge more modular and interpretable, a latent knowledge retriever is used during pre-training, fine-tuning and inference.
3. REALM (Retrieval-Augmented Language Model Pre-Training) outperforms previous methods by a significant margin on three popular Open-QA benchmarks, while also providing qualitative benefits such as interpretability and modularity.
The article is generally trustworthy and reliable in its claims. The authors provide evidence for their claims in the form of experiments conducted on three popular Open-QA benchmarks, showing that REALM outperforms previous methods by a significant margin. The article does not appear to be one-sided or biased in any way; it presents both sides of the argument equally and provides evidence for each claim made. There are no unsupported claims or missing points of consideration; all relevant information is provided in the article. Furthermore, there is no promotional content or partiality present in the article; it simply presents the facts without any bias or opinionated language. Finally, possible risks are noted throughout the article; for example, the authors note that larger networks may be required to cover more facts due to implicit knowledge storage in neural networks parameters. In conclusion, this article is trustworthy and reliable with regards to its claims and presentation of information.