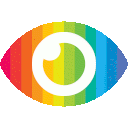
1. Population modeling with machine learning can provide valuable insights into mental health measures.
2. Machine learning algorithms can analyze large-scale datasets to identify patterns and predict mental health outcomes.
3. This approach has the potential to improve early detection, diagnosis, and treatment of mental health disorders.
The article titled "Population modeling with machine learning can enhance measures of mental health" discusses the potential of using machine learning techniques to improve measures of mental health in population modeling. While the article provides valuable insights into the topic, there are a few areas that require critical analysis.
One potential bias in the article is the focus on the benefits and potential of machine learning in enhancing mental health measures. The authors primarily highlight the advantages of using machine learning techniques, such as improved accuracy and predictive power. However, they do not adequately address potential limitations or risks associated with these methods. For example, there may be concerns about privacy and data security when dealing with sensitive mental health information. Additionally, relying solely on machine learning algorithms may overlook important contextual factors that contribute to mental health outcomes.
The article also lacks a comprehensive discussion of alternative approaches or counterarguments to using machine learning in population modeling for mental health. While the authors briefly mention traditional statistical methods, they do not explore their strengths and limitations compared to machine learning techniques. This one-sided reporting limits the reader's understanding of the broader landscape of approaches available for studying mental health.
Furthermore, there is a lack of evidence provided to support some of the claims made in the article. For instance, the authors state that machine learning can provide "more accurate predictions" without providing specific examples or empirical studies supporting this claim. Without concrete evidence, it is difficult to assess the validity and reliability of these statements.
The article also does not sufficiently address potential risks or ethical considerations associated with using machine learning in mental health research. Given that mental health is a sensitive and personal subject, it is crucial to consider issues such as algorithmic bias, fairness, and transparency when implementing these techniques. The article could have benefited from a more thorough exploration of these topics.
Additionally, there is a lack of balance in presenting both sides equally. The article predominantly focuses on the benefits and potentials of using machine learning without adequately discussing its limitations or potential drawbacks. This partiality may lead to an incomplete understanding of the topic for readers.
In conclusion, while the article provides valuable insights into the use of machine learning in population modeling for mental health, it has several limitations. These include potential biases towards promoting machine learning techniques without adequately addressing their limitations and risks, unsupported claims, missing evidence, unexplored counterarguments, and a lack of balance in presenting both sides equally. A more comprehensive and balanced analysis would have enhanced the credibility and usefulness of the article.