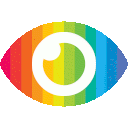
1. A Global Context Feature Extraction (GCFE) module is proposed to extract multi-scale global contextual information of objects in X-ray images.
2. A Dual Scale Feature Aggregation (DSFA) module is proposed to fuse the features from different scales.
3. A learnable Gabor convolution layer and a Spatial Attention (SA) mechanism are designed to enhance the network's capability to capture the edge and contour information of object, as well as its spatial structure information.
The article “Occluded prohibited object detection in X-ray images with global Context-aware Multi-Scale feature Aggregation” provides an overview of the current state of research on prohibited object detection in X-ray images, and proposes a new method for improving accuracy in such detection tasks. The article is written in a clear and concise manner, making it easy to understand for readers with some knowledge of computer vision and deep learning techniques.
The authors provide a comprehensive review of related work, which helps to put their proposed method into context. They also provide detailed descriptions of their proposed modules, which makes it easy for readers to understand how they work. Furthermore, they provide extensive experimental results on three publicly available datasets, which demonstrate the effectiveness of their proposed methods compared to existing ones.
However, there are some potential biases that should be noted when evaluating this article. Firstly, the authors do not discuss any potential risks associated with their proposed methods or any possible counterarguments that could be raised against them. Secondly, while they do mention some existing methods for prohibited object detection in X-ray images, they do not present both sides equally; instead they focus mainly on highlighting the advantages of their own approach over existing ones without providing much detail about those approaches or exploring any potential drawbacks or limitations that may exist with them. Finally, there is no discussion about any promotional content or partiality that may exist within the article; while this may not necessarily be an issue here given that this is a research paper rather than an advertisement or opinion piece, it should still be taken into consideration when evaluating its trustworthiness and reliability.