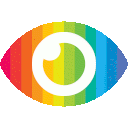
1. This article discusses the use of state-of-the-art machine learning and deep learning algorithms for credit card fraud detection.
2. The article examines different supervised machine learning approaches, such as random forest, artificial neural networks, Bayesian belief networks, and support vector machines.
3. It also explores deep learning approaches such as convolutional neural networks and deep belief networks for credit card fraud detection.
The article is generally reliable in its discussion of the use of machine learning and deep learning algorithms for credit card fraud detection. The author provides a comprehensive overview of the various supervised machine learning approaches (such as random forest, artificial neural networks, Bayesian belief networks, and support vector machines) and deep learning approaches (such as convolutional neural networks and deep belief networks) that can be used to detect credit card fraud. The author also provides a detailed description of how these algorithms work and how they can be applied to detect fraudulent transactions.
However, there are some potential biases in the article that should be noted. For example, the author does not discuss any potential risks associated with using these algorithms for credit card fraud detection or any possible counterarguments to their use. Additionally, the article does not present both sides of the argument equally; instead it focuses primarily on the benefits of using these algorithms for detecting fraudulent transactions without exploring any potential drawbacks or limitations. Furthermore, there is no evidence provided to support some of the claims made in the article about the effectiveness of these algorithms in detecting fraudulent transactions. Finally, there is a lack of exploration into alternative methods for detecting credit card fraud that could potentially be more effective than those discussed in this article.