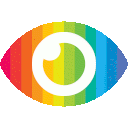
1. The proposed multi-modal learning framework integrates focal tumor image, tumor geometrics, and global brain network features derived from MRI to predict the genotype of glioma.
2. The framework includes separate encoders for each modality, a self-supervised approach to generate brain networks from anatomical MRI, a hierarchical attention module for feature extraction, and a bi-level multi-modal contrastive loss to align features and tackle the domain gap.
3. Experimental results show that the proposed model outperforms baseline deep learning models in predicting glioma genotype, and the visualized interpretation corresponds to clinical knowledge.
The article titled "Multi-Modal Learning for Predicting the Genotype of Glioma" presents a novel approach for predicting the genotype of glioma, a type of brain tumor. The authors propose a multi-modal learning framework that integrates focal tumor image, tumor geometrics, and global brain network features derived from MRI. They use convolutional neural networks (CNN) to predict the isocitrate dehydrogenase (IDH) gene mutation, which is an essential biomarker for the diagnosis and prognosis of glioma.
The article provides a comprehensive overview of the current methods used for predicting glioma genotypes, including radiomics-based machine learning approaches and deep learning models. It highlights the limitations of these approaches and argues that CNNs cannot effectively learn from non-Euclidean data such as geometric and network data. The authors propose their multi-modal learning framework as a solution to this problem.
One potential bias in the article is that it focuses primarily on the benefits and advantages of their proposed method without adequately discussing its limitations or potential risks. While they mention that their model outperforms baseline deep learning models in experimental results, they do not provide detailed information about the performance metrics or any potential drawbacks or challenges associated with their approach.
Another potential bias is that the article does not present alternative viewpoints or counterarguments to their proposed method. It assumes that integrating multi-modal data is inherently beneficial for predicting glioma genotypes without considering any potential drawbacks or limitations of this approach.
Additionally, the article lacks specific evidence or references to support some of its claims. For example, it states that MRI can predict glioma genotypes using machine learning or deep learning models with a unique non-invasive advantage over conventional invasive approaches. However, no specific studies or evidence are provided to support this claim.
Furthermore, while the article mentions that diffusion MRI is limited in availability and proposes a self-supervised approach to generate brain networks from anatomical MRI, it does not discuss the potential limitations or challenges of using anatomical MRI alone for constructing brain networks.
Overall, the article presents an interesting approach for predicting the genotype of glioma using multi-modal learning. However, it has some potential biases and lacks specific evidence or discussion of limitations and risks associated with the proposed method. Further research and validation are needed to fully assess the effectiveness and feasibility of this approach in clinical practice.