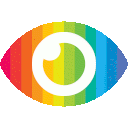
1. Cellular senescence is a fundamental process in aging and is increasingly recognized as a target for pharmaceutical intervention.
2. Nuclear morphology can be used to assess cellular senescence with computer vision and machine learning methods.
3. Deep learning models can accurately predict cellular senescence based on nuclear morphology, which correlates with markers of senescence such as SA-β-gal, p16Ink4a, p21Cip1, and p53.
The article “Nuclear Morphology is a Deep Learning Biomarker of Cellular Senescence | Nature Aging” provides an overview of the use of deep learning models to predict cellular senescence based on nuclear morphology. The authors present evidence that their model is accurate in predicting senescent cells from normal cells and that it correlates with markers of senescence such as SA-β-gal, p16Ink4a, p21Cip1, and p53.
The article appears to be well researched and the authors provide evidence for their claims. However, there are some potential biases that should be noted. For example, the authors focus primarily on the positive aspects of using deep learning models to predict cellular senescence without exploring any potential risks or drawbacks associated with this approach. Additionally, the article does not explore any counterarguments or alternative approaches to predicting cellular senescence which could provide a more balanced view of the topic.
Furthermore, while the authors do provide evidence for their claims regarding accuracy and correlation with markers of senescence, they do not provide any evidence for their claims regarding how predicted senescence relates to human disease or cancer risk. This lack of evidence makes it difficult to assess the trustworthiness and reliability of these claims.
In conclusion, while this article provides an interesting overview of using deep learning models to predict cellular senescence based on nuclear morphology, there are some potential biases that should be noted including lack of exploration into potential risks or drawbacks associated with this approach as well as lack of evidence for certain claims made by the authors regarding human disease or cancer risk.