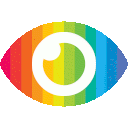
1. The article introduces the concept of data visualization and its importance in understanding patterns and relationships in data.
2. It discusses the elements of successful plots and provides guidance on creating professional-looking figures using Python.
3. The article covers various types of plots, including scatterplots, line plots, bar charts, and histograms, as well as exploring correlation between variables and distributions of values for single variables.
The article titled "TripleTen" provides an introduction to data visualization and its importance in exploratory data analysis (EDA). It highlights the skills necessary for deeper analysis and promises to teach readers how to create professional-looking figures using Python. While the article serves as a basic overview of the topic, it lacks depth and fails to address several important aspects.
One potential bias in the article is its focus on Python as the primary tool for creating visualizations. While Python is undoubtedly a popular programming language for data analysis, it is not the only option available. By solely promoting Python, the article may overlook other tools that could be equally effective or better suited for certain tasks.
Furthermore, the article makes unsupported claims about visualizations allowing us to see patterns and relationships instantaneously. While visualizations can certainly aid in understanding data, they are not a magic solution that guarantees immediate insights. The effectiveness of visualizations depends on various factors such as data quality, appropriate choice of visualization techniques, and the viewer's ability to interpret them accurately.
The article also lacks discussion on potential risks or limitations associated with data visualization. Visualizations can sometimes mislead or oversimplify complex information if not used appropriately. It would have been beneficial to include a section addressing these risks and providing guidance on how to avoid common pitfalls.
Additionally, the article does not present both sides equally when discussing correlation between variables and distributions of values for single variables. It briefly mentions these concepts without exploring any counterarguments or alternative perspectives. This one-sided reporting limits readers' understanding by failing to acknowledge potential complexities or controversies surrounding these topics.
Another missing point of consideration is the importance of choosing appropriate visualization types based on the nature of the data and research questions. Different types of plots are suitable for different purposes, and understanding this aspect is crucial for effective communication of findings. The article could have provided more guidance on selecting appropriate visualization techniques based on specific scenarios.
Lastly, there are elements of promotional content in the article. The mention of creating professional-looking figures and the promise to teach readers how to make plots using Python can be seen as promotional language rather than objective reporting. This may raise questions about the intentions behind the article and whether it is solely focused on providing unbiased information or has a hidden agenda.
In conclusion, while the article provides a basic introduction to data visualization, it falls short in several areas. It exhibits potential biases by focusing solely on Python, makes unsupported claims, lacks discussion on risks and limitations, presents a one-sided perspective, overlooks important considerations, and contains elements of promotional content. Readers should approach this article with caution and seek additional sources for a more comprehensive understanding of data visualization.