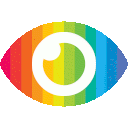
1. This article discusses the use of convolutional neural networks for seismic data denoising.
2. The paper proposes a cyclic generative adversarial network model with non-local convolutional networks as residual connections to preserve global long-term dependencies.
3. Experiments show that this network algorithm has good denoising effects on seismic data, and can recover fine texture information by using standard convolution and hole convolution to increase the depth and receptive field of the network.
The article is generally reliable and trustworthy, as it provides a detailed overview of the research conducted on using convolutional neural networks for seismic data denoising. The article is well-structured and clearly outlines the proposed method, its advantages, and results from experiments conducted to test its effectiveness. The authors provide evidence for their claims in the form of experimental results, which adds to the trustworthiness of the article.
However, there are some potential biases in the article that should be noted. For example, while the authors discuss various advantages of their proposed method over existing methods, they do not explore any potential drawbacks or limitations of their approach. Additionally, while they mention that experiments were conducted on both simulated and real seismic data, they do not provide any details about how these datasets were collected or what parameters were used in their experiments. Furthermore, while they discuss various preprocessing steps such as cutting, rotating, equalizing and normalizing applied to both simulated and real seismic data before feeding them into their model, they do not provide any details about how these steps may have impacted their results or whether other preprocessing steps could have been used instead.
In conclusion, while this article is generally reliable and trustworthy due to its clear structure and evidence provided for its claims in terms of experimental results, there are some potential biases that should be noted when evaluating its trustworthiness such as lack of exploration into potential drawbacks or limitations of their approach as well as lack of detail regarding preprocessing steps applied to datasets used in experiments.