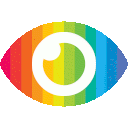
1. Traumatic brain injury (TBI) is a growing public health hazard caused by various types of head impacts from different sources, and brain strain is an effective biomechanical predictor for TBI/mTBI injury risk.
2. A machine-learning-based classifier using spectral densities of linear acceleration and angular velocity was built to classify head impact types, reaching a median accuracy of 96% over 1000 random partitions of training and test sets.
3. Type-specific nearest-neighbor regression models were developed for three injury risk metrics with classification, showing higher R2 values than the baseline model without subtyping. The classifier enables a better understanding of the impact kinematics spectral density in different sports and can be applied to evaluate the quality of impact-simulation systems and on-field data augmentation.
该文章是一篇关于机器学习在头部撞击分类中的应用的研究。文章提到了头部撞击对脑损伤的影响以及不同类型头部撞击对脑损伤风险的差异性,同时探讨了机器学习在分类和预测脑损伤风险方面的应用。
然而,该文章存在一些潜在偏见和局限性。首先,文章只考虑了机械因素对脑损伤的影响,而忽略了其他因素如个体差异、生理状态等可能对结果产生影响的因素。其次,文章没有充分考虑到数据来源的可靠性和代表性问题。例如,实验室重建数据与真实场景下头部撞击可能存在差异,这可能会导致模型预测结果出现偏差。
此外,在研究方法上也存在一些问题。例如,文章没有详细说明分类器选择和参数设置的依据,并且没有进行交叉验证等方法来评估模型效果。此外,在解释特征重要性时也缺乏足够的解释和论证。
最后,该文章还存在一定程度上宣传内容和偏袒之嫌。例如,在介绍TBI/mTBI危害时,文章强调了其高死亡率和高治疗成本,但没有提及其他类型的脑损伤可能也存在类似的问题。此外,在介绍机器学习分类器时,文章只强调了其优点而忽略了可能存在的风险和局限性。
因此,该文章需要更加全面和客观地考虑问题,并在方法和结果解释上进行更加严谨的论证。