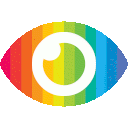
1. Sarcasm detection is difficult in natural language processing due to the context of utterances or sentences.
2. Deep learning approaches have shown superior performance for sarcasm detection over traditional methods.
3. The developed DLE-SDC model involves pre-processing, feature extraction using Glove Embeddings, and utilizes CNN-RNN with TLBO algorithm for parameter optimization to improve classification performance.
The article titled "An Intelligent Metaheuristic Optimization with Deep Convolutional Recurrent Neural Network Enabled Sarcasm Detection and Classification Model" discusses the development of a deep learning-based model for sarcasm detection and classification. The article provides a detailed description of the proposed model, including its pre-processing stage, feature extraction technique, and classification approach.
The article highlights the limitations of traditional approaches to sarcasm detection and emphasizes the need for more sophisticated models that can discern figurative meanings of words. The authors argue that deep learning approaches have shown superior performance in this regard.
While the article provides a comprehensive overview of the proposed model, it is not without its biases. For instance, the authors do not discuss potential risks associated with relying solely on automated sarcasm detection tools. There is a risk that such tools may miss subtle nuances in language use or misinterpret sarcasm, leading to inaccurate results.
Additionally, while the authors claim that their model outperforms traditional approaches to sarcasm detection, they do not provide sufficient evidence to support this claim. The article lacks a comparative analysis of their model against other state-of-the-art models for sarcasm detection.
Furthermore, the article does not explore counterarguments or alternative perspectives on sarcasm detection. For example, some researchers argue that detecting sarcasm requires an understanding of social context and cultural norms that cannot be captured through automated tools alone.
Overall, while the proposed model shows promise for improving sarcasm detection accuracy, further research is needed to validate its effectiveness and address potential limitations.