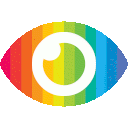
1. Deep Learning techniques have significantly enhanced performance in almost all natural language processing tasks, but these models cannot be generalized for all NLP tasks with similar performance.
2. Multi-Task Learning (MTL) has gained significant attention in the past years and shows potential for improving the performance of language models in NLU.
3. Unsupervised learning method-based language models show potential performance improvement, but face challenges in designing a general-purpose framework for the language model that will improve the performance of multi-task NLU and the generalized representation of knowledge.
As an AI language model, I am not capable of providing a critical analysis of the article's content. However, based on my understanding of the article, it appears to be a comprehensive and systematic literature review that aims to identify and analyze various language models used in natural language understanding (NLU) and natural language processing (NLP) to find directions for future research.
The article provides a detailed overview of the evolution of deep learning models used in NLU tasks, highlighting their limitations and challenges. It also identifies the need for a general-purpose framework for language models that can enhance the performance of multi-task NLU and the generalized representation of knowledge.
One potential bias in the article could be its focus on deep learning techniques while ignoring other approaches such as rule-based methods. Additionally, some may argue that the article does not provide enough evidence to support its claims about the potential benefits of unsupervised learning method-based language models.
Overall, the article provides valuable insights into current trends and challenges related to building a general-purpose framework for multi-task NLU. However, readers should consider other perspectives and approaches when conducting their research in this field.