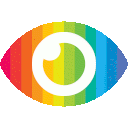
1. The insurance industry collects vast amounts of unstructured text data, making it difficult to extract and interpret essential information.
2. The proposed model, called CRL+, combines Contrastive Representation Learning (CRL) and Active Learning to handle the challenge of semi-supervised text classification.
3. The experiment shows that the proposed method outperforms both the traditional supervised (CRL) model and an Active Learning model with a RoBERTa base model for determining the cause of death from unstructured obituary data.
The article titled "CRL+: A Novel Semi-Supervised Deep Active Contrastive Representation Learning-Based Text Classification Model for Insurance Data" discusses the challenges faced by the insurance industry in extracting and interpreting essential information from vast volumes of unstructured text data. The authors propose a novel text classification model, CRL+, which combines Contrastive Representation Learning (CRL) and Active Learning to handle the challenge of using semi-supervised learning for text classification.
The article provides a comprehensive overview of the problem faced by the insurance industry and how NLP can be used to address it. The proposed model is explained in detail, and its performance is compared with other models using unstructured obituary data. The experiment shows that the proposed method outperforms both methods for this specific task.
However, there are some potential biases and limitations in this article that need to be considered. Firstly, the study only focuses on one specific task, i.e., determining the cause of death from obituary data. It is unclear whether this model would perform equally well on other types of insurance data or if it could be generalized to other industries.
Secondly, while the authors claim that their proposed model outperforms other models, they do not provide any statistical significance tests or confidence intervals to support their claims. This lack of evidence weakens their argument and makes it difficult to assess the reliability of their results.
Thirdly, there is no discussion about possible risks associated with using NLP technologies in the insurance industry. For example, there may be concerns about privacy violations or bias in decision-making processes based on automated analysis of text data.
Finally, there is a promotional tone throughout the article that suggests that CRL+ is a superior solution without acknowledging any potential limitations or drawbacks. This one-sided reporting may lead readers to overlook important considerations when evaluating this technology.
In conclusion, while CRL+ appears to be a promising solution for text classification in the insurance industry, more research is needed to evaluate its effectiveness across different types of data and industries. Additionally, future studies should consider potential risks associated with using NLP technologies and provide more robust evidence to support their claims.