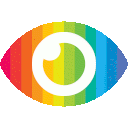
1. The article proposes Pathomic Fusion, an integrated framework for fusing histology and genomic features for cancer diagnosis and prognosis.
2. The approach models pairwise feature interactions across modalities using the Kronecker product of unimodal feature representations and controls the expressiveness of each representation via a gating-based attention mechanism.
3. The proposed multimodal fusion paradigm improves prognostic determinations from ground truth grading and molecular subtyping, as well as unimodal deep networks trained on histology and genomic data alone.
The article titled "Pathomic Fusion: An Integrated Framework for Fusing Histopathology and Genomic Features for Cancer Diagnosis and Prognosis" presents a novel approach for integrating histology image and genomic data to improve cancer diagnosis, prognosis, and therapeutic response prediction. The authors propose a multimodal fusion strategy called Pathomic Fusion, which combines histology image features extracted using Convolutional Neural Networks (CNNs) with genomic features such as mutations, copy number variations (CNV), and RNA sequencing (RNA-Seq) data.
The article highlights the limitations of current deep learning-based prediction models that rely on either histology or genomics alone and do not effectively utilize the complementary information from both modalities. The authors argue that by fusing these modalities in an intuitive manner, they can improve prognostic determinations and better understand diseases.
One potential bias in the article is the lack of discussion on the limitations of using deep learning models for cancer diagnosis and prognosis. While deep learning has shown promise in various medical applications, including histopathology analysis, it is important to acknowledge that these models are not infallible and may have limitations in terms of generalizability, interpretability, and potential biases in training data.
Another potential bias is the focus on the proposed Pathomic Fusion framework as a superior approach compared to previous methods. While the authors provide evidence from their experiments using glioma and clear cell renal cell carcinoma datasets, it would be beneficial to compare their results with other state-of-the-art methods in the field to provide a more comprehensive evaluation.
Additionally, there is limited discussion on potential risks or challenges associated with integrating histology image and genomic data. For example, issues related to data quality, standardization across different laboratories or sequencing platforms, and privacy concerns should be addressed when considering the implementation of such multimodal fusion approaches in clinical settings.
Furthermore, while the article mentions that interpretability is an important aspect of their proposed framework, there is limited discussion on how the interpretability of the fused multimodal features is achieved. The authors briefly mention the use of class-activation maps and gradient-based attribution techniques, but more details and examples would be helpful in understanding how these methods contribute to the interpretability of the model.
Overall, while the article presents an interesting approach for integrating histology image and genomic data for cancer diagnosis and prognosis, there are some biases and limitations that should be considered. Further research and evaluation are needed to validate the effectiveness and generalizability of the proposed Pathomic Fusion framework in diverse cancer types and datasets.