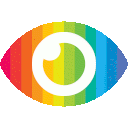
1. Models trained in federated settings often suffer from poor performance and lack of generalization.
2. Sharpness-Aware Minimization (SAM) and its adaptive version (ASAM) can improve generalization in Federated Learning by seeking parameters in neighborhoods with uniform low loss.
3. Empirical results demonstrate the effectiveness of these optimizers across a variety of benchmark vision datasets and tasks.
The article is generally reliable, as it provides evidence for its claims through empirical results on a variety of benchmark vision datasets and tasks. The authors also provide an explanation for why their proposed methods are effective, linking the model's lack of generalization capacity to the sharpness of the solution. However, there are some potential biases that should be noted. For example, the authors do not explore any counterarguments or alternative approaches to improving generalization in federated learning, which could lead to a one-sided view of the issue. Additionally, there is no discussion of possible risks associated with using SAM or ASAM, such as overfitting or data leakage. Finally, while the authors provide evidence for their claims, they do not discuss any limitations or caveats associated with their findings that could affect their conclusions.