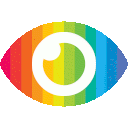
1. The article discusses the use of ensemble-based machine learning approaches in QSAR prediction for drug discovery to overcome constraints and obtain reliable predictions.
2. The proposed comprehensive ensemble method outperformed thirteen individual models on 19 bioassay datasets and demonstrated superiority over other ensemble approaches that are limited to a single subject.
3. The article introduces a multi-subject diversified ensemble method, a novel neural network-based individual classifier, and second-level meta-learning for combining models, providing an interpretation of the importance of individual models through their learned weights.
The article titled "Comprehensive ensemble in QSAR prediction for drug discovery" published in BMC Bioinformatics discusses the use of ensemble-based machine learning approaches in quantitative structure-activity relationship (QSAR) modeling for drug discovery. The authors highlight the constraints and challenges associated with traditional QSAR modeling, such as a large number of chemicals, sparse data, and errors in datasets. They argue that ensemble learning, which combines multiple models to improve predictive power, can overcome these limitations.
One potential bias in the article is the focus on promoting the proposed comprehensive ensemble method as superior to other existing approaches. While the authors claim that their method outperformed thirteen individual models on 19 bioassay datasets, they do not provide a detailed comparison with other ensemble methods or discuss potential drawbacks or limitations of their approach. This one-sided reporting may lead readers to believe that the proposed method is the best option without considering alternative perspectives.
Additionally, the article lacks evidence or examples to support some of its claims. For instance, while the authors mention that neural networks have gained attention in QSAR prediction following a Kaggle competition in 2012, they do not provide specific results or references to validate this statement. Providing more concrete evidence and references would strengthen the credibility of their arguments.
Furthermore, the article does not explore potential counterarguments or alternative viewpoints regarding ensemble learning in QSAR prediction. It would be beneficial for readers to understand different perspectives on this topic and consider potential drawbacks or challenges associated with ensemble methods.
The promotional tone of the article is also evident in its emphasis on highlighting the strengths and advantages of the proposed method without acknowledging any possible risks or limitations. It is essential for researchers and practitioners in drug discovery to be aware of both the benefits and drawbacks of different approaches to make informed decisions.
In conclusion, while the article presents an interesting approach to improving QSAR prediction through comprehensive ensemble learning, it could benefit from addressing potential biases by providing a more balanced discussion of alternative methods, supporting claims with evidence, exploring counterarguments, and acknowledging possible risks or limitations. By doing so, readers can gain a more comprehensive understanding of the topic and make informed decisions in their research endeavors.