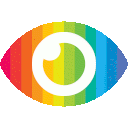
1. Photovoltaic (PV) generation is a widely used renewable energy source, but faults in PV systems can lead to significant losses and safety hazards.
2. Detection and classification of PV faults are crucial for preventing system failures and minimizing losses.
3. Various methods, including visual/thermal methods, electrical-based methods, and artificial intelligence techniques, are used for fault detection and classification in PV systems.
The article titled "Diagnosis of PV faults using digital twin and convolutional mixer with LoRa notification system" provides an overview of the detection and classification of faults in photovoltaic (PV) systems. While the article presents valuable information on the topic, there are several areas that require critical analysis.
One potential bias in the article is the emphasis on electrical-based methods (EBMs) for fault detection and classification. The author states that EBMs attract more attention than visual/thermal methods (VTMs) due to their versatility and wide range of applications. However, this claim lacks supporting evidence or a balanced discussion of the advantages and disadvantages of both approaches. It would have been beneficial to explore the limitations of EBMs and highlight cases where VTMs may be more suitable.
Additionally, the article mentions that ML and DL models have been proven to be effective and accurate in detecting and classifying PV faults. While this statement may be true, it lacks specific examples or references to studies that support this claim. Without concrete evidence, it is difficult to assess the reliability and generalizability of these models.
Furthermore, the article does not adequately address potential risks associated with PV faults. While it briefly mentions that faults can lead to revenue loss, panel damage, and even fires, it does not delve into the potential consequences in detail. A more comprehensive analysis would have included discussions on safety hazards, environmental impacts, and economic implications.
The article also lacks exploration of counterarguments or alternative perspectives. It primarily focuses on the use of AITs for fault detection without considering other approaches or methodologies. Including a broader range of viewpoints would have provided a more balanced analysis.
Moreover, there are instances where claims are made without sufficient evidence or support. For example, when discussing different AITs used for fault classification, the author mentions specific algorithms such as Naïve Bayes and logistic regression without providing any justification for their effectiveness in this context.
Additionally, the article does not address potential limitations or challenges in implementing the proposed digital twin and convolutional mixer with LoRa notification system. It would have been valuable to discuss factors such as cost, scalability, and feasibility of implementation.
Overall, while the article provides a general overview of PV fault detection and classification, it lacks depth in certain areas and fails to provide a balanced analysis. The biases towards EBMs and AITs, unsupported claims, missing evidence, and lack of consideration for alternative perspectives limit the overall credibility and reliability of the article.