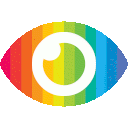
1. The growth of big data and AI has led to an increase in information facets, such as equivocality and veracity, which can significantly influence human perceptions of information and affect performance.
2. There is a need for artificially intelligent technologies that can adapt information representations to overcome cognitive limitations in complex information environments.
3. The Adaptive Cognitive Fit (ACF) framework proposes using AI to augment and improve human performance by dynamically evolving the representation of information to improve functional affordances and symbolic value.
The article "Adaptive cognitive fit: Artificial intelligence augmented management of information facets and representations" discusses the impact of big data and artificial intelligence (AI) on human cognition and performance. The authors argue that the increasing complexity and diversity of information representations, coupled with the rise of information facets such as equivocality and veracity, necessitate the development of AI technologies that can adapt information representations to overcome cognitive limitations.
The article provides a comprehensive review of extant research on cognitive fit, which focuses on aligning information representation with task to optimize human performance. However, the authors argue that this approach is insufficient in the context of big data and AI ecosystems, where information facets play a dominant role in shaping human perception and decision-making.
The authors propose a novel framework called Adaptive Cognitive Fit (ACF), which explains how AI-augmented information representations can improve human performance by adapting to cognitive limitations imposed by information facets. They draw on information processing theory and cognitive dissonance theory to develop four ACF propositions, which they empirically validate through an economic experiment and a machine learning simulation.
Overall, the article presents a compelling argument for the need to develop AI technologies that can adapt to complex information environments. However, there are several potential biases and limitations in the article that should be noted.
Firstly, the article focuses primarily on the benefits of AI-augmented information representations without adequately considering potential risks or drawbacks. For example, there may be concerns about privacy violations or unintended consequences arising from biased algorithms.
Secondly, while the authors acknowledge that weak levels of information facets may influence individuals differently, they do not fully explore how individual differences in expertise or knowledge may interact with dominant information facets to shape perception and decision-making.
Thirdly, while the empirical validation of ACF through an economic experiment and machine learning simulation is promising, it is unclear how generalizable these findings are across different domains or contexts.
Finally, there is some promotional content in the article, particularly in the introduction and conclusion, which may overstate the potential benefits of AI technologies for improving human performance.
In conclusion, while the article provides a valuable contribution to the literature on cognitive fit and AI-augmented information representations, it is important to consider its potential biases and limitations when interpreting its findings. Further research is needed to fully understand how AI can be used to adapt information representations to overcome cognitive limitations imposed by information facets.