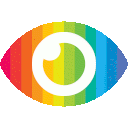
1. The study aimed to identify the particles that better discriminate industries regarding potential negative effects on workers’ health due to occupational exposure to particulate matter.
2. Six different methods of discriminant analysis were applied and compared for classification accuracy, with artificial neural networks yielding the highest rate of accuracy.
3. Results indicate that the particle number concentration of diameter size 0.5 µm is the parameter that better discriminates industries in terms of potential negative health effects on workers.
The article titled "Comparison of discriminant analysis methods: Application to occupational exposure to particulate matter" presents a study on the health effects associated with occupational exposure to particulate matter in six industries. The study aims to identify the particles that better discriminate the industries, with the ultimate goal of classifying industries regarding potential negative effects on workers’ health.
The article compares several methods of discriminant analysis, including linear discriminant analyses (LDA), linear quadratic discriminant analysis (QDA), robust linear discriminant analysis with selected estimators (MLE, MVE, “t”, MCD, MCD-A, MCD-B), multinomial logistic regression and artificial neural networks (ANN). The predictive accuracy of these methods was accessed through a simulation study.
The results indicate that ANN yielded the highest rate of classification accuracy in the data set under study. However, there are some potential biases and limitations in this study. For instance, the sample size is relatively small as only six industries were selected for analysis. Additionally, the focus on particle number concentration for six different diameters may not be sufficient to fully capture all potential negative health effects associated with occupational exposure to particulate matter.
Furthermore, while the article acknowledges that certain environmental conditions in animal and plant production have been associated with increased frequency in respiratory illnesses among farmers occupationally exposed in swine production, it does not explore potential counterarguments or alternative explanations for these findings.
Overall, while this article provides valuable insights into different methods of discriminant analysis for identifying potential negative health effects associated with occupational exposure to particulate matter, it is important to consider its limitations and potential biases when interpreting its findings.