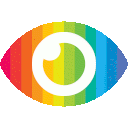
1. The number of grains per panicle is an important trait for rice cultivation and variety screening, but current manual counting methods are labor-intensive and time-consuming.
2. This study developed an image analysis-based method using linear regression and deep learning algorithms to accurately count the number of grains per panicle in rice, even when grains are touching or overlapping.
3. This method has potential for high-throughput counting of grain number per panicle in other crops, improving efficiency in scientific research and cultivar development.
The article "Image analysis-based recognition and quantification of grain number per panicle in rice" presents a new method for counting the number of grains per panicle in rice using image processing and deep learning algorithms. The authors compare the accuracy of their method with other existing methods and demonstrate that their approach is more accurate, cost-effective, and user-friendly.
The article provides a comprehensive background on the importance of phenotyping in plant research and the challenges associated with manual methods for obtaining phenotypic data. The authors highlight the significance of grain number per panicle as a key trait affecting grain cultivation, management, and yield, and emphasize the need for rapid measurement of this trait to improve scientific research and cultivar development.
The authors discuss various image-based methods that have been used to measure different phenotypic traits in rice, including plant height, leaf area, tiller number, biomass prediction, nitrogen and water status assessment, and seed morphology characterization. They also describe the limitations of existing methods for counting grain number per panicle due to overlapping or clustered grains.
The authors propose a new method that uses digital scanners to acquire images of primary branches without stems or noise. They then apply image pre-processing techniques such as Otsu segmentation algorithm, connected area extraction, erosion-dilation operations to remove noise and stem from binary images. Finally, they use linear regression or deep learning algorithms to count the number of grains per panicle based on feature parameters such as coverage degree (CD), skeleton (Sk), contour (Co).
While the article provides detailed information about the methodology used by the authors to develop their new method for counting grain number per panicle in rice, it has some potential biases that need to be considered. For example:
- The study only focuses on two varieties each of Indica and Japonica subspecies of rice grown under specific seeding density and fertilizer conditions. Therefore, it may not be applicable to other varieties or species grown under different conditions.
- The authors do not provide any information about potential risks associated with using digital scanners or image processing algorithms for plant phenotyping.
- The article does not explore any counterarguments against their proposed method or compare it with other recently developed methods.
- While the authors claim that their approach is more accurate than existing methods for counting grain number per panicle in rice, they do not provide enough evidence to support this claim.
- The article seems promotional at times when discussing how their proposed method can be a huge asset for guiding high throughput methods for counting grain number per panicle in other crops.
In conclusion, while "Image analysis-based recognition and quantification of grain number per panicle in rice" presents an innovative approach for counting grain number per panicle using image processing techniques and deep learning algorithms; it has some potential biases that need to be considered before applying this method widely. Further studies are needed to validate its accuracy across different varieties/species grown under varying conditions.