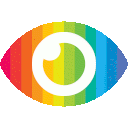
1. The article presents a deep learning model called TransPhos for predicting phosphorylation sites in proteins, which is essential for various biological processes.
2. TransPhos outperforms several other deep learning models and state-of-the-art prediction tools in general protein phosphorylation site prediction.
3. The model achieves good performance on training datasets of Serine, Threonine, and Tyrosine, demonstrating its effectiveness in predicting different types of phosphorylation sites.
The article titled "TransPhos: A Deep-Learning Model for General Phosphorylation Site Prediction Based on Transformer-Encoder Architecture" presents a novel deep learning-based predictor for phosphorylation site prediction. While the article provides valuable information about the importance of protein phosphorylation and the challenges in predicting phosphorylation sites, there are several aspects that need critical analysis.
One potential bias in the article is the lack of discussion on the limitations and potential risks associated with using deep learning models for phosphorylation site prediction. Deep learning models heavily rely on training data, and if the training data is biased or incomplete, it can lead to inaccurate predictions. The article does not mention how the training data was collected or if any biases were addressed during model development.
Another issue is that the article claims that their model outperforms several deep learning models and state-of-the-art prediction tools without providing sufficient evidence to support this claim. The results section mentions that TransPhos performs better than other models in terms of AUC and MCC, but there is no detailed comparison or statistical analysis provided. Without proper evaluation metrics and statistical analysis, it is difficult to assess the true performance of TransPhos compared to other methods.
Additionally, the article lacks a comprehensive discussion on potential counterarguments or alternative approaches to phosphorylation site prediction. It would have been beneficial to explore different methodologies and compare their strengths and weaknesses. This would provide readers with a more balanced view of the field and help them understand the limitations of TransPhos.
Furthermore, there is a lack of transparency regarding the dataset used for training and testing TransPhos. The article mentions two databases (PPA and Phospho. ELM) but does not provide details about these datasets or how they were curated. Without this information, it is challenging to assess whether the dataset represents a diverse range of phosphorylation sites or if it introduces any biases into the model.
The article also lacks discussion on potential risks or limitations of using TransPhos in real-world applications. For example, it does not address the challenges of applying the model to different species or the generalizability of the predictions. This information is crucial for researchers and practitioners who may consider using TransPhos in their work.
In terms of promotional content, the article heavily promotes TransPhos as a superior predictor without providing sufficient evidence to support this claim. The language used throughout the article is biased towards highlighting the strengths of TransPhos while downplaying the limitations or uncertainties associated with its performance.
Overall, while the article presents an interesting deep learning-based predictor for phosphorylation site prediction, it lacks critical analysis, transparency, and comprehensive evaluation. It would benefit from addressing potential biases, providing more evidence for its claims, discussing alternative approaches, and acknowledging limitations and potential risks associated with using TransPhos.