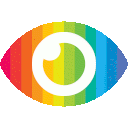
1. This study evaluated the prognostic value of computed tomography (CT) analysis using deep learning algorithms in patients with chronic hepatitis B (CHB).
2. The study found that deep learning-based measurements of spleen volume, visceral adipose tissue (VAT), and subcutaneous adipose tissue (SAT) indices were significant predictors for various outcomes such as hepatocellular carcinoma (HCC) development, hepatic decompensation, diabetes mellitus (DM), and overall survival.
3. These findings suggest that CT analysis using deep learning algorithms can provide valuable prognostic information for patients with CHB.
The article titled "Prognostic role of computed tomography analysis using deep learning algorithm in patients with chronic hepatitis B viral infection" discusses the use of deep learning algorithms to analyze computed tomography (CT) scans in patients with chronic hepatitis B (CHB) and evaluate their prognostic value. While the study provides valuable insights into the potential use of CT analysis for predicting clinical outcomes in CHB patients, there are several points that need to be critically analyzed.
One potential bias in this study is the retrospective design, which may introduce selection bias and confounding factors. The authors included 2,169 patients with CHB who underwent contrast-enhanced abdominal CT for hepatocellular carcinoma (HCC) surveillance. However, it is unclear how these patients were selected and whether they represent a diverse population. This lack of information raises concerns about the generalizability of the findings.
Another potential bias is related to the use of deep learning algorithms for CT analysis. While these algorithms have shown promise in various medical applications, including image segmentation and disease diagnosis, they are not without limitations. The article does not discuss the potential limitations or sources of error associated with deep learning algorithms used in this study. It would be important to address issues such as algorithm training data quality, overfitting, and generalizability to different patient populations.
Furthermore, the article does not provide detailed information on how the prognostic models were developed or validated. It is unclear whether external validation was performed to assess the generalizability of the models beyond the study cohort. Without proper validation, it is difficult to determine whether the models can accurately predict clinical outcomes in other CHB patient populations.
The article also lacks a discussion on potential confounding factors that may influence the relationship between CT measurements and clinical outcomes. For example, it is well-known that liver fibrosis stage plays a crucial role in determining disease progression and prognosis in CHB patients. However, the article does not mention whether liver fibrosis stage was considered in the analysis or how it may interact with the CT measurements.
Additionally, the article focuses primarily on the positive findings of the study, such as significant predictors of HCC, hepatic decompensation, diabetes mellitus (DM), and overall survival. However, it does not discuss any negative findings or limitations of the study. This one-sided reporting may create an overly optimistic view of the potential utility of CT analysis in CHB patients.
Moreover, there is a lack of discussion on potential risks or harms associated with CT analysis using deep learning algorithms. While CT scans are generally considered safe, they do involve exposure to ionizing radiation, which carries a small but non-negligible risk of cancer development. The article does not address this risk or discuss any measures taken to minimize radiation exposure in the study cohort.
In conclusion, while the article provides interesting insights into the potential use of CT analysis using deep learning algorithms for predicting clinical outcomes in CHB patients, there are several limitations and biases that need to be critically analyzed. The retrospective design, lack of detailed information on algorithm performance and validation, missing consideration of confounding factors, one-sided reporting, and absence of discussion on potential risks are some key points that should be addressed to fully evaluate the validity and generalizability of the study findings.