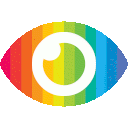
1. Tigrinya is a low-resource language with limited linguistic materials and tools, making it challenging to develop effective automated classification systems for Tigrinya text.
2. Convolutional neural networks (CNNs) have gained popularity in natural language processing research, and this study explores the use of CNNs for Tigrinya text classification using word embedding techniques such as FastText and word2vec.
3. The study contributes two Tigrinya datasets, including a single-label dataset and a large unlabeled corpus, and evaluates the performance of various word embedding architectures and CNNs for classifying Tigrinya news articles. The results show that word2vec significantly improves classification accuracy, outperforming other approaches by 93.41%.
The article titled "Text Classification Based on Convolutional Neural Networks and Word Embedding for Low-Resource Languages: Tigrinya" presents a study on the use of convolutional neural networks (CNNs) and word embedding techniques for text classification in the low-resource language of Tigrinya. The authors highlight the challenges of working with Tigrinya due to its complex morphological structure, lack of data resources, and underdeveloped linguistic tools. They also note the increasing importance of Tigrinya textual data on the internet and the need for an effective automated classification system.
The article provides a comprehensive review of previous attempts at natural language processing (NLP) in Tigrinya, highlighting the lack of research using neural network approaches for text classification. The authors also discuss conventional text classification frameworks and their various stages, including preprocessing, feature extraction, feature selection, and classification.
One potential bias in this article is that it focuses solely on CNNs and word embedding techniques for text classification in Tigrinya. While these methods have shown promising results in other languages, there may be other approaches that could be equally or more effective in Tigrinya. Additionally, the authors do not explore potential limitations or drawbacks of using CNNs and word embedding techniques.
Another limitation is that the study only evaluates two types of word embedding models (FastText and word2vec) and does not compare them to other models or techniques. This narrow focus may limit the generalizability of their findings.
The article also lacks discussion on potential ethical considerations related to NLP research in low-resource languages such as Tigrinya. For example, there may be concerns around data privacy or cultural sensitivity when working with textual data from marginalized communities.
Overall, while this article provides valuable insights into using CNNs and word embedding techniques for text classification in low-resource languages like Tigrinya, it would benefit from a more balanced discussion of alternative approaches and potential limitations or ethical considerations.