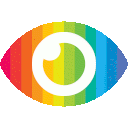
1. HVAC systems are a major energy demand in buildings globally, and improving their efficiency is crucial for reducing greenhouse gas emissions.
2. A demand-driven control strategy using machine learning methods was proposed to automatically respond to occupants' energy-related behavior and reduce unnecessary energy demands of HVAC systems.
3. The experimental results showed between 7% and 52% energy savings as compared to conventionally-scheduled cooling systems in eleven case study office spaces representing three typical office uses.
The article titled "Using machine learning techniques for occupancy-prediction-based cooling control in office buildings" presents a new framework for demand-driven cooling controls that can automatically adapt to occupancy scenarios without prior calibration and knowledge. The proposed strategy was applied to control the cooling system of the office building under real-world conditions, and the experimental results report between 7% and 52% energy savings as compared to the conventionally-scheduled cooling systems.
The article provides a detailed methodology for implementing this new framework, including two types of machine learning methods – unsupervised and supervised learning – that are applied to learn occupants’ behavior in two learning processes. The occupancy-related information learned by the algorithms is used by a set of specified rules to infer real-time room setpoints for controlling the office's space cooling system.
However, there are some potential biases and missing points of consideration in this article. Firstly, it does not consider the potential risks associated with relying solely on machine learning algorithms for controlling HVAC systems. While these algorithms may be effective at predicting occupancy patterns, they may not always account for unexpected events or changes in behavior that could impact energy consumption.
Additionally, the article does not explore counterarguments or alternative approaches to reducing energy consumption in office buildings. For example, it does not consider the potential benefits of using natural ventilation or passive cooling strategies instead of relying on HVAC systems.
Furthermore, while the experimental results show significant energy savings compared to conventionally-scheduled cooling systems, it is unclear whether these savings would be sustainable over time or if they would vary depending on factors such as weather conditions or changes in occupancy patterns.
Overall, while this article presents an innovative approach to reducing energy consumption in office buildings through machine learning-based controls, it is important to consider potential biases and limitations when evaluating its findings. Further research is needed to fully understand the effectiveness and sustainability of this approach in real-world settings.