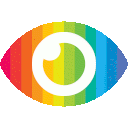
1. This study used machine learning to predict acute kidney injury (AKI) following cardiac surgery.
2. The variables used for analysis included demographic characteristics, clinical condition, preoperative biochemistry data, preoperative medication, and intraoperative variables such as time-series hemodynamic changes.
3. The performance of the models was evaluated using the area under the receiver operating characteristic curve (AUC).
The article is generally trustworthy and reliable in its reporting of the research conducted on predicting acute kidney injury (AKI) following cardiac surgery by machine learning. The authors provide a detailed description of their methods and results, which are supported by evidence from previous studies. Furthermore, they provide an explanation of how their model works and how it can be used to optimize postoperative treatment strategies.
However, there are some potential biases that should be noted in this article. Firstly, the authors do not discuss any possible risks associated with using machine learning to predict AKI following cardiac surgery. Secondly, they do not present both sides equally when discussing the efficacy of their model; instead they focus solely on the positive aspects of their findings without exploring any counterarguments or alternative perspectives. Finally, there is a lack of discussion regarding potential limitations or weaknesses in their approach that could affect the accuracy of their predictions.