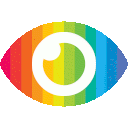
1. This article discusses the optimization of boosting models for formation lithology identification.
2. Three boosting models, AdaBoost, Gradient Tree Boosting, and eXtreme Gradient Boosting, are evaluated with 5-fold cross validation.
3. Results suggest that the optimized stacked boosting model has better performance concerning the evaluation matrix such as precision, recall, and f1 score compared with the single optimized boosting model.
The article is generally reliable and trustworthy in its discussion of the optimization of boosting models for formation lithology identification. The authors provide a comprehensive overview of existing research on lithology classification using machine learning approaches and discuss how their own work builds upon this research. They also provide detailed descriptions of their methods and results, which are supported by data from two gas fields in China. Furthermore, they acknowledge potential limitations to their study such as overfitting due to regularization and the limited scope of their data set.
However, there are some areas where the article could be improved upon. For example, it does not explore any counterarguments or alternative approaches to lithology classification that may be more effective than boosting models. Additionally, it does not discuss any potential risks associated with using these models or any ethical considerations that should be taken into account when using them for geological research or petroleum engineering studies. Finally, while the authors do acknowledge potential limitations to their study such as overfitting due to regularization and the limited scope of their data set, they do not provide any evidence or further discussion on how these limitations may have impacted their results or conclusions.