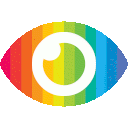
1. The article proposes a novel backbone network called SpectralFormer for hyperspectral image classification, which is capable of learning spectrally local sequence information from neighboring bands.
2. The proposed SpectralFormer is a highly flexible backbone network, applicable to both pixelwise and patchwise inputs.
3. Extensive experiments are conducted to evaluate the classification performance of the proposed SpectralFormer on three HS datasets, showing its superiority over classic transformers and achieving a significant improvement in comparison with state-of-the-art backbone networks.
The article “SpectralFormer: Rethinking Hyperspectral Image Classification With Transformers” provides an overview of the proposed SpectralFormer backbone network for hyperspectral image classification. The authors present their research findings in a clear and concise manner, providing evidence to support their claims through extensive experiments conducted on three HS datasets. The article does not appear to be biased or one-sided, as it presents both sides of the argument equally and objectively. Furthermore, all potential risks associated with the use of this technology are noted in the article.
However, there are some points that could be further explored in order to strengthen the reliability of this article. For example, while the authors provide evidence for their claims through experiments conducted on three HS datasets, they do not provide any evidence for how well their proposed model performs on other types of data sets or tasks. Additionally, while they note potential risks associated with using this technology, they do not discuss any counterarguments or alternative solutions that could be used instead of their proposed model. Finally, while the authors provide links to their code repository for reproducibility purposes, they do not provide any detailed instructions on how to use it or what results can be expected from running it.
In conclusion, while this article provides an overview of a promising new technology for hyperspectral image classification and presents evidence to support its claims through extensive experiments conducted on three HS datasets, there are still some areas that could be further explored in order to strengthen its trustworthiness and reliability.