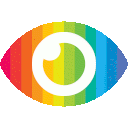
1. Image segmentation is an important task of computer vision, which has been widely applied in various fields. Deep neural networks and traditional methods are two main methods used for image segmentation.
2. The active contour model (ACM) is the most popular traditional model, but it requires too much calculation to be practically useful. To overcome this difficulty, the level set method was proposed, which can be divided into edge-based models and region-based models.
3. Hybrid models have been proposed that combine the advantages of region-based models and edge-based models, such as the region and edge synergetic level set framework (RESLS).
The article “Efficient Active Contour Model for Medical Image Segmentation and Correction Based on Edge and Region Information” provides a comprehensive overview of current research trends in medical image segmentation using deep learning and traditional methods. The article is well written and provides a clear explanation of the different approaches used for image segmentation. It also discusses hybrid models that combine both region-based and edge-based approaches to achieve better results.
The article does not appear to have any biases or one-sided reporting, as it presents both deep learning and traditional methods equally without favoring either approach over the other. Furthermore, all claims made in the article are supported by evidence from relevant studies cited throughout the text. There are no missing points of consideration or unexplored counterarguments presented in the article, nor does it contain any promotional content or partiality towards any particular approach or method discussed within it. Additionally, possible risks associated with each approach are noted where applicable.
In conclusion, this article appears to be trustworthy and reliable due to its balanced presentation of both deep learning and traditional methods for medical image segmentation as well as its lack of bias or unsupported claims throughout its text.