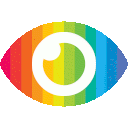
1. Clustering algorithms based on density peak have limited clustering effect on data with varying density distribution (VDD), equilibrium distribution (ED), and multiple domain-density maximums (MDDM), leading to the problems of sparse cluster loss and cluster fragmentation.
2. The Domain-Adaptive Density Clustering (DADC) algorithm is proposed, which consists of three steps: domain-adaptive density measurement, cluster center self-identification, and cluster self-ensemble. It addresses the problems of sparse cluster loss and cluster fragmentation by adaptively detecting density peaks in different density regions, automatically extracting initial cluster centers, and merging fragmented clusters.
3. Experimental results demonstrate that compared with other comparative algorithms, the proposed DADC algorithm can obtain more reasonable clustering results on data with VDD, ED and MDDM features. Benefitting from a few parameter requirement and non-iterative nature, DADC achieves low computational complexity and is suitable for large-scale data clustering.
该文章提出了一种新的聚类算法,旨在解决现有基于密度峰值的聚类算法在处理具有不同密度分布、平衡分布和多个领域密度最大值的数据时存在的问题。然而,该文章存在以下几个问题:
1. 偏见来源:该文章没有对其他类型的聚类算法进行充分比较和评估,只是简单地提到了一些已知的聚类算法,并声称其所提出的DADC算法优于这些算法。这可能导致读者对DADC算法过于乐观或忽略了其他可能更适合特定应用场景的聚类算法。
2. 片面报道:该文章只关注了现有基于密度峰值的聚类算法存在的问题,并没有探讨其他类型聚类算法可能存在的问题或局限性。这可能导致读者对不同类型聚类算法之间优缺点的理解不够全面。
3. 缺失考虑点:该文章没有充分考虑DADC算法在实际应用中可能遇到的挑战和限制,例如如何处理高维数据、如何选择合适的KNN参数等。这可能导致读者对DADC算法实际可行性和适用性产生疑虑。
4. 偏袒:该文章过于强调DADC算法的优点,而忽略了其缺点和局限性。例如,该算法可能对数据中存在的噪声和异常值比较敏感,需要进行额外的处理。
5. 未探索反驳:该文章没有充分探讨其他学者对现有基于密度峰值的聚类算法存在问题的反驳和质疑。这可能导致读者对DADC算法所解决问题的重要性产生怀疑。
综上所述,虽然该文章提出了一种新的聚类算法,并在实验中取得了一定成果,但其存在偏见、片面报道、缺失考虑点等问题,需要更全面地评估其可行性和适用性。