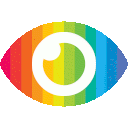
1. Amyotrophic lateral sclerosis (ALS) is a rare primary neurodegenerative syndrome with no cure, but early intervention can improve life quality and survival.
2. Genetic variants delivered by genome-wide association studies (GWAS) only account for 10% of the heritability of ALS, leaving 40% unaccounted for.
3. Capsule networks have been proposed as a potential remedy to address this issue, as they are capable of classifying distorted or entangled patterns in images correctly and require less training data than convolutional neural networks.
The article “Predicting the prevalence of complex genetic diseases from individual genotype profiles using capsule networks | Nature Machine Intelligence” provides an overview of the current state of research on predicting the prevalence and occurrence of Amyotrophic Lateral Sclerosis (ALS). The article is written in a clear and concise manner, providing an accessible introduction to the topic for readers who may not be familiar with it. The authors provide a comprehensive overview of the challenges associated with predicting ALS prevalence, including the need to account for missing heritability and non-additive genetic effects such as epistasis. They then discuss how deep learning approaches such as convolutional neural networks (CNNs) have been used to address these challenges, but have faced criticisms due to their lack of interpretability and high demand for training data. Finally, they propose capsule networks (CapsNets) as a potential solution due to their ability to classify distorted or entangled patterns in images correctly while requiring less training data than CNNs.
The article is generally well-written and provides an informative overview of the current state of research on predicting ALS prevalence. However, there are some areas where it could be improved upon. For example, while the authors provide an overview of how CNNs have been used to address these challenges, they do not provide any evidence that CapsNets are actually more effective than CNNs in this regard. Additionally, while they mention that CapsNets enable “a human-mind-friendly interpretation” of results, they do not provide any evidence or examples that demonstrate this claim. Furthermore, while they mention several studies that have modelled gene–gene interactions when predicting ALS prevalence, they do not discuss any potential limitations or drawbacks associated with these approaches. Finally, while they mention several applications of deep learning when predicting phenotype from genotype profiles, they do not discuss any potential ethical implications associated with using such approaches in clinical settings.
In conclusion, this article provides an informative overview of the current state of research on predicting ALS prevalence using deep learning approaches such as capsule networks (CapsNets). However, it could be improved upon by providing more evidence for its claims and discussing potential limitations or drawbacks associated with existing approaches as well as ethical implications associated with using deep learning in clinical settings.