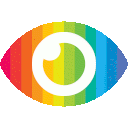
1. A machine learning model was developed to predict acute respiratory distress syndrome (ARDS) in patients with sepsis in the ICU using clinical data, with ten critical features identified including PaO2/PAO2, A-aDO2, and CRP.
2. Among eight different algorithms tested, the Gaussian NB algorithm performed significantly better in predicting ARDS in patients with sepsis.
3. The developed machine learning model showed good performance in training, validation, and test sets, with an AUC of 0.781 and accuracy of 78.6%, providing a more accurate prediction compared to previous models.
The article titled "Machine learning for the early prediction of acute respiratory distress syndrome (ARDS) in patients with sepsis in the ICU based on clinical data" presents a study that aims to develop a machine-learning model for predicting ARDS in patients with sepsis. The study utilizes initial clinical data of patients with sepsis admitted to the hospital to predict ARDS and identify crucial variables.
One potential bias in this study could be the selection of variables used for prediction. While the authors mention screening out crucial variables, there may be other important factors that were not considered in the analysis. Additionally, the choice of algorithms used for comparison could introduce bias, as certain algorithms may perform better on specific types of data.
The article lacks discussion on potential limitations of using machine learning models for predicting ARDS in patients with sepsis. Machine learning models are only as good as the data they are trained on, and there may be limitations in the quality or quantity of clinical data available for prediction. Furthermore, there is no mention of potential risks associated with relying solely on a machine learning model for clinical decision-making.
The article also does not provide insights into potential ethical considerations when using machine learning models in healthcare settings. There may be concerns about privacy, transparency, and accountability when implementing predictive models in clinical practice.
Moreover, the article does not explore counterarguments or alternative approaches to predicting ARDS in patients with sepsis. It would have been beneficial to discuss other methods or tools that could complement or validate the findings of the machine learning model.
Overall, while the study presents interesting findings regarding the early prediction of ARDS in patients with sepsis using machine learning, there are several areas where further exploration and discussion are needed to provide a comprehensive analysis of the topic.