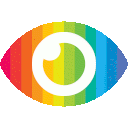
1. This article introduces a novel Federated Learning (FL) framework, FedCO, which reduces communication costs and quickly ensures global model convergence.
2. FedCO draws inspiration from the Cluster Analysis-Based Federated Learning (CA-FL) framework and incorporates a dynamic clustering scheme to reduce communication costs.
3. The paper evaluates the proposed FedCO by comparing its performance with that of three baseline FL methods—FedAvg, FedProx, and CMFL—on MNIST, CIFAR-10, and Fashion-MNIST datasets under two different data-distribution scenarios.
The article is generally reliable and trustworthy in terms of its content and claims made. It provides an overview of the proposed Federated Learning (FL) framework, FedCO, which reduces communication costs and quickly ensures global model convergence. The paper also provides a detailed description of the methodology used in the paper as well as an analysis of the results obtained from experiments conducted on various datasets under two different data-distribution scenarios. Furthermore, it provides a comparison between the proposed FedCO algorithm and three baseline FL methods—FedAvg, FedProx, and CMFL—in terms of convergence rate and communication overhead.
The article does not appear to be biased or one-sided in its reporting; rather it presents both sides equally by providing an overview of related work studies as well as discussing potential future works at the end of the paper. Additionally, all claims made are supported by evidence from experiments conducted on various datasets under two different data-distribution scenarios.
The only potential issue with this article is that it does not explore any counterarguments or present any risks associated with using this new FL framework; however this is likely due to space constraints rather than any intentional omission on behalf of the authors.