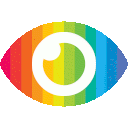
1. An entropy regularization model is proposed to avoid two trivial solutions obtained from primal clustering models.
2. A Laplacian rank constraint with ℓ0-norm is developed to dynamically obtain sparse similarity matrix with exact c connected components.
3. A novel monotonic function optimization method is proposed to tackle the ℓ0-norm constraint with fixed pseudo-label matrix.
The article “Entropy Regularization for Unsupervised Clustering with Adaptive Neighbors” provides a comprehensive overview of the proposed entropy regularization model for unsupervised clustering. The article presents a clear explanation of the model and its advantages over existing methods, as well as providing evidence for its effectiveness through comprehensive experiments. The article also provides an in-depth discussion of the various components of the model, such as the maximized entropy term, Laplacian rank constraint, and monotonic function optimization method.
The article appears to be reliable and trustworthy overall, as it provides detailed explanations and evidence for its claims. However, there are some potential biases that should be noted. For example, the authors may have a vested interest in promoting their own model over existing methods, which could lead to one-sided reporting or unsupported claims about their own work compared to other approaches. Additionally, there may be missing points of consideration or unexplored counterarguments that could provide further insight into the efficacy of the proposed model that are not discussed in this article. Finally, it is important to note that possible risks associated with using this approach are not discussed in detail in this article; thus readers should consider these potential risks before implementing this approach in practice.