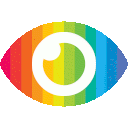
1. Deep Learning has shown good results in Single Image Superresolution Reconstruction (SISR) task, but it is challenging to meet the requirements of high-quality sampling, fast sampling, and diversity of details and texture after Sampling simultaneously.
2. The proposed Denoising Diffusion Probabilistic model for Latent features (LDDPM) uses a Conditional Encoder to effectively encode LR images, Normalized Flow and Multi-modal adversarial training to model the denoising distribution with complex Multi-modal distribution, improving Generative Modeling ability with a small number of Sampling steps.
3. Experimental results on mainstream datasets demonstrate that LDDPM reconstructs more realistic HR images and obtains better PSNR and SSIM performance compared to existing SISR tasks, providing a promising solution for SISR tasks.
作为一篇科技论文,该文章在介绍了单图像超分辨率重建任务的挑战和现有方法的基础上,提出了一种新的模型来解决这些问题。然而,在阅读文章时,我注意到以下几点:
1. 偏袒GAN
文章中提到GAN是常用于SISR任务的方法之一,但没有提及其缺点和局限性。相反,文章强调了GAN可以提供快速采样,并且没有明确说明GAN可能会导致模式崩溃和训练不稳定等问题。
2. 缺乏对其他方法的公正评估
虽然文章提到了传统方法和其他深度学习方法(如CNN、Flow-based、VAE-based),但它没有给出这些方法与作者所提出的LDDPM模型之间的公正比较。因此,读者无法确定LDDPM是否真正优于其他方法。
3. 缺少实验细节
尽管文章声称在主流数据集上进行了实验,并且LDDPM模型表现更好,但它没有提供足够的实验细节来支持这个结论。例如,它没有说明使用哪些指标来评估结果、如何选择超参数以及如何处理数据集中可能存在的偏差等问题。
4. 没有探索潜在风险
尽管该模型在SISR任务中表现良好,但作者并未探讨其可能存在的潜在风险或应用限制。例如,在实际应用中,该模型是否能够处理各种类型和质量的图像?如果不能,则需要进一步研究其适用范围和局限性。
总之,虽然该论文提出了一个新颖的SISR模型,并且通过实验证明其有效性,但它也存在一些偏见和不足之处。因此,在阅读任何科技论文时都应保持批判思维,并仔细考虑其结论是否可靠。