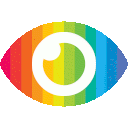
1. Big Data and multi-source information systems: The article discusses the challenges posed by the massive amount of data generated in the age of Big Data, which is produced by multiple sources with high velocity, volume, and variety. It highlights the need for effective methods to process multi-source information systems or datasets.
2. Granular computing (GrC) as a useful method for information fusion: The article argues that granular computing, a rapidly developing information processing paradigm, is the most useful method for research on Big Data. GrC is based on Zadeh's concept of "information granularity" and involves using granules in problem-solving processes. It has been applied in various fields such as fuzzy sets and rough set theory.
3. Information fusion techniques: The article explores the concept of information fusion, which involves integrating multi-source information for signal processing, image processing, knowledge representation, and inference. Various techniques such as Bayesian networks, evidence theory, and mixture aggregation operators have been used for information fusion. The article also mentions specific applications of information fusion in areas like drillability prediction and fault diagnosis.
Overall, the article emphasizes the importance of effectively processing multi-source datasets in the age of Big Data and highlights granular computing as a valuable approach for information fusion. It also provides insights into different techniques used for information fusion in various domains.
对于上述文章的批判性分析如下:
1. 潜在偏见及其来源:文章中存在潜在的偏见,主要体现在对大数据和多源信息融合方法的过度宣扬。作者将大数据描述为“无与伦比的资源”,并声称多源信息融合是处理大数据最有效的方法,但没有提供充分的证据来支持这一观点。这种偏见可能源自于作者对自己研究领域的过度推崇和宣传。
2. 片面报道:文章只关注了多源信息融合方法中的粒计算视角,而忽略了其他可能存在的方法和视角。这种片面报道可能导致读者对该领域的理解不全面,并丧失了探索其他潜在方法的机会。
3. 无根据的主张:文章声称粒计算是处理大数据最有效的方法,但没有提供充分的证据来支持这一主张。缺乏实证研究或案例分析来验证粒计算在多源信息融合中的优势。
4. 缺失的考虑点:文章没有充分考虑到多源信息融合中可能存在的挑战和问题。例如,如何解决不同数据源之间的异构性、一致性和可信度等问题,并确保融合结果的准确性和可靠性。
5. 所提出主张的缺失证据:文章中提到了粒计算在信息处理中的一些应用案例,但没有提供充分的证据来支持这些案例。缺乏实证研究或实验数据来验证粒计算在多源信息融合中的有效性。
6. 未探索的反驳:文章没有探讨可能存在的反驳观点或争议。例如,是否有其他方法可以更好地处理大数据和多源信息融合问题?是否存在其他领域对此进行了深入研究并取得了重要成果?
7. 宣传内容和偏袒:文章过度宣扬粒计算方法在多源信息融合中的优势,而忽略了其他可能存在的方法和视角。这种宣传内容可能导致读者对该领域的理解产生误导,并丧失了客观评估不同方法之间优劣的机会。
8. 是否注意到可能的风险:文章没有明确提及可能存在的风险或潜在问题。例如,在大数据时代,隐私保护和数据安全是一个重要问题,但文章没有对此进行讨论。
9. 没有平等地呈现双方:文章只关注粒计算方法在多源信息融合中的优势,而没有平等地呈现其他可能存在的方法和视角。这种不平等的呈现可能导致读者对该领域的理解产生偏差。
综上所述,上述文章存在潜在偏见、片面报道、无根据的主张、缺失的考虑点、所提出主张的缺失证据、未探索的反驳、宣传内容和偏袒等问题。读者在阅读该文章时应保持批判思维,并寻找更多来源和证据来全面了解大数据和多源信息融合领域。