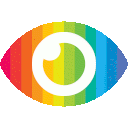
1. The article compares the performance of a used Xeon E5 machine build to a newer i7-7820X build for deep learning.
2. The Xeon E5 build offers advantages such as lower cost, the option for multiple CPUs, and support for multiple GPUs.
3. Recommendations are provided for those on a budget or looking for a high-performance multi-thread, multi-GPU rig.
The article titled "New vs used deep-learning machine builds, Part 2" provides a detailed comparison between a used Xeon E5 machine build and a newer i7-7820X build for deep learning purposes. While the article offers some useful information, there are several areas where it lacks critical analysis and presents biased or unsupported claims.
Firstly, the author emphasizes the importance of researching a solid platform to plug the GPU into but fails to provide any evidence or examples to support this claim. It would have been helpful to include specific cases or scenarios where choosing the right platform made a significant difference in performance or functionality.
Additionally, the article focuses heavily on the technical specifications and performance metrics of the two builds but fails to consider other important factors such as power consumption, scalability, and compatibility with different software frameworks. These factors can greatly impact the overall usability and cost-effectiveness of a deep learning machine.
Furthermore, the author only recommends Intel CPUs over AMD without providing any justification or explanation for this bias. It would have been more balanced to present both options and discuss their respective strengths and weaknesses.
The article also lacks exploration of counterarguments or alternative perspectives. For example, while it compares boot times and CPU performance between the two builds, it does not address potential trade-offs in terms of power consumption or heat generation. This omission limits the reader's ability to make an informed decision based on their specific needs and constraints.
Moreover, there is a lack of evidence or data supporting some of the claims made in the article. For instance, when comparing NVME vs SSD performance, the author states that there would be no difference in training time on GPU based on reading weights at start and writing out weights at end. However, no evidence is provided to support this claim.
Finally, there is a promotional tone throughout the article, particularly when discussing specific products such as Noctua fans and Thermaltake fan controllers. This raises questions about the author's potential biases and whether they have a vested interest in promoting these products.
In conclusion, while the article provides some useful information about comparing new and used deep learning machine builds, it lacks critical analysis, presents biased or unsupported claims, and fails to consider important factors beyond technical specifications. Readers should approach the information with caution and seek additional sources to make an informed decision.