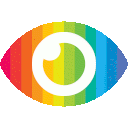
1. The article presents a novel approach to constructing pipeline feature engineering for AutoML services, which simplifies the development of machine learning applications.
2. The approach involves expanding the feature space sequentially and using autoencoders to reduce input feature dimensions and reconstruct output features.
3. Experimental results on VANET network traffic data show that the proposed Feature Engineering approach improves the accuracy of machine learning models, with the highest classification accuracy achieved at 91.2% using a Multi-layered Model autoencoder and XGBoost classification model.
The article titled "Development of pipeline feature engineering for building an AutoML service" presents a new approach to constructing pipeline feature engineering for an AutoML (Automatic Machine Learning) service. The aim of this approach is to simplify the development of machine learning applications by expanding the feature space and using autoencoders to reduce dimensionality.
One potential bias in this article is the lack of discussion on the limitations and challenges of using AutoML. While the article mentions that one limitation of implementing artificial intelligence approaches is the availability of research competencies, it does not address other potential limitations such as data quality issues, interpretability of models, and ethical considerations. This omission may give readers a skewed perspective on the capabilities and drawbacks of AutoML.
Additionally, the article lacks a thorough explanation of the methodology used in constructing the pipeline feature engineering. It briefly mentions sequential expansion of the feature space and the use of autoencoders but does not provide details on how these techniques are implemented or their theoretical underpinnings. This lack of information makes it difficult for readers to assess the validity and reliability of the proposed approach.
Furthermore, while the article presents results from experiments conducted on VANET network traffic data, it does not provide sufficient evidence or statistical analysis to support its claims about the effectiveness of using the proposed Feature Engineering approach. The claim that machine learning models on source data have an average accuracy 2% lower than those using Feature Engineering is unsupported without further analysis or comparison with alternative methods.
The article also lacks exploration of counterarguments or alternative approaches to pipeline feature engineering. It presents only one approach based on autoencoders without discussing other possible techniques or their potential advantages/disadvantages. This narrow focus limits readers' understanding and evaluation of different strategies for feature engineering in AutoML.
Moreover, there is a promotional tone throughout the article, emphasizing the effectiveness and superiority of the presented Feature Engineering approach without acknowledging any potential drawbacks or limitations. This biased presentation raises questions about the objectivity and impartiality of the research.
In terms of potential risks, the article does not explicitly note any risks associated with using AutoML or the proposed Feature Engineering approach. It is important to consider and discuss potential risks such as model bias, privacy concerns, and unintended consequences when implementing automated machine learning systems.
Overall, this article lacks critical analysis, provides limited evidence for its claims, and presents a biased perspective on the effectiveness of pipeline feature engineering in AutoML. It would benefit from a more comprehensive discussion of limitations, alternative approaches, and potential risks associated with using AutoML.