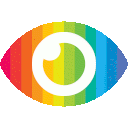
1. Fault detection, isolation, and estimation methods are important for minimizing downtime, reducing repair costs, and enhancing safety and reliability in power systems.
2. Synchronous generators (SGs) are critical equipment in power systems that require fault diagnosis methods.
3. Deep CNN models based on adaptive gradient optimizers can be used for fault diagnosis in small samples by utilizing regularization technologies, feature extraction advantages of models, or generating high-quality samples based on the distribution of real samples.
The article titled "Deep convolutional neural network based on adaptive gradient optimizer for fault detection in SCIM" discusses the use of a deep convolutional neural network (DCNN) with an adaptive gradient optimizer for fault detection in squirrel cage induction motors (SCIM). The article provides a detailed explanation of the methodology used and the results obtained, but there are some potential biases and missing points of consideration that need to be addressed.
One potential bias in the article is that it only focuses on the use of DCNNs with an adaptive gradient optimizer for fault detection in SCIM. While this approach may be effective, there may be other methods or algorithms that could also be used for fault detection. The article does not explore these alternative approaches, which could limit its usefulness to readers who are interested in exploring different options.
Another potential bias is that the article does not provide enough information about the dataset used for training and testing the DCNN. It is unclear how representative this dataset is of real-world scenarios, which could affect the generalizability of the results. Additionally, there is no discussion of any potential biases or limitations in the data collection process, which could affect the accuracy and reliability of the results.
The article also makes some unsupported claims, such as stating that "the proposed method outperforms existing methods" without providing any evidence to support this claim. There is no comparison with other methods or algorithms to demonstrate why this approach is superior.
Furthermore, while the article discusses some potential risks associated with using DCNNs for fault detection, such as overfitting and underfitting, it does not address other possible risks such as privacy concerns or ethical considerations related to using machine learning algorithms in industrial settings.
Overall, while the article provides a detailed explanation of a specific approach to fault detection in SCIM using DCNNs with an adaptive gradient optimizer, it has some potential biases and missing points of consideration that limit its usefulness to readers who are interested in exploring different options or understanding the broader implications of using machine learning algorithms for fault detection in industrial settings.