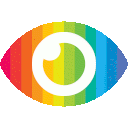
1. The article presents a comparative study of three vessel segmentation algorithms in terms of accuracy, sensitivity, and specificity on two publicly available datasets.
2. The simulations indicate that the frangi and Otsu thresholding based algorithm yields the highest accuracy on the DRIVE dataset, while the Unet-based convolutional neural network algorithm outperforms the others on the STARE dataset.
3. The study provides valuable insights for physicians and surgeons in choosing an appropriate algorithm for images with complex structures, such as palm print lines or computer tomography.
Based on the content of the article, it appears to be a comparative study of three vessel segmentation algorithms. The study aims to evaluate the accuracy, sensitivity, and specificity of these algorithms on two publicly available datasets.
The article starts by highlighting the importance of vessel segmentation in the timely treatment of various diseases. It mentions that automated segmentation algorithms can provide improved results compared to manual segmentation and can save time. The authors state that their main contributions are a detailed comparison of three recently developed algorithms and an investigation into the effects of image resolution on algorithm performance.
The article then provides a brief overview of recent developments in vessel segmentation algorithms, mentioning various techniques such as retinal vessel segmentation, fuzzy c-means clustering, deep learning models, and morphological hessian-based methods.
Next, the article describes the three compared vessel segmentation algorithms in detail. Algorithm A is an integrated SUACE and Tyler Coye based method, Algorithm B uses Frangi filter and Otsu thresholding, and Algorithm C is based on Unet convolutional neural networks. The article provides equations and explanations for each algorithm's operation.
In the simulation results section, the authors mention that they ran simulations on a high-performance workstation using DRIVE and STARE datasets. They explain that these datasets contain images marked by human observers and are divided into training and test sets. The evaluation metrics used are accuracy, sensitivity, and specificity.
Overall, the article provides a comprehensive overview of vessel segmentation algorithms and presents simulation results comparing their performance. However, there are some potential biases or limitations to consider:
1. Limited scope: The study only compares three specific vessel segmentation algorithms. There may be other algorithms or techniques that were not included in this analysis.
2. Dataset selection: The choice of datasets may introduce bias as they are limited to DRIVE and STARE datasets. Other datasets with different characteristics could yield different results.
3. Lack of generalizability: The study does not provide information about the generalizability of the algorithms to other datasets or real-world scenarios.
4. Lack of statistical analysis: The article does not mention any statistical analysis or significance testing for the results. It is unclear if the observed differences in performance are statistically significant.
5. Missing evidence: The article does not provide detailed evidence or examples to support its claims about the performance of each algorithm. It would be helpful to see visual comparisons or specific examples of segmentation results.
6. Unexplored counterarguments: The article does not discuss potential limitations or drawbacks of the compared algorithms. It would be valuable to explore any known challenges or limitations associated with each algorithm.
7. Promotional content: The article does not appear to have any promotional content, as it is a technical study comparing algorithms rather than promoting a specific product or service.
In conclusion, while the article provides a detailed comparison of vessel segmentation algorithms, there are some potential biases and limitations that should be considered. Further research and analysis would be needed to validate and generalize the findings of this study.