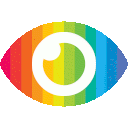
1. The article proposes a deep clustering convolutional autoencoder model to effectively cluster histopathology images of cholangiocarcinoma based on similar morphologies.
2. The model combines a neural network-based clustering cost-function with a novel deep convolutional architecture to produce clusters relevant to patient outcome.
3. The performance and usefulness of the clustering model are assessed through survival analysis, which measures the correlation between each cluster of histomorphologies and cancer recurrence after resection.
The article titled "Unsupervised Subtyping of Cholangiocarcinoma Using a Deep Clustering Convolutional Autoencoder" discusses the use of a deep learning technique to cluster histopathology images of cholangiocarcinoma, a type of cancer. The authors propose combining a neural network-based clustering cost function with a deep convolutional architecture to effectively cluster the large amount of high intra-variance data into subsets based on similar morphologies.
One potential bias in this article is the lack of discussion about the limitations and potential risks associated with using unsupervised clustering techniques for subtyping cancer. While the authors mention that other studies have used similar techniques for image segmentation, they do not address the challenges and potential pitfalls of relying solely on unsupervised methods for clinical decision-making. It would be important to consider factors such as inter-observer variability, reproducibility, and generalizability when applying these clustering algorithms to real-world patient outcomes.
Additionally, the article does not provide sufficient evidence or references to support some of its claims. For example, the authors state that their proposed clustering model will produce clusters of histopathology relevant to patient outcome, but there is no clear explanation or evidence provided to support this claim. Without proper validation and external validation studies, it is difficult to determine if these clusters are truly meaningful in predicting patient outcomes.
Furthermore, the article lacks discussion on potential confounding factors that may influence the clustering results and subsequent survival analysis. Factors such as tumor stage, grade, and molecular characteristics could significantly impact patient outcomes but are not mentioned or accounted for in this study. It is important to consider these variables when interpreting the results and drawing conclusions about the relationship between histomorphologies and recurrence of cancer after resection.
The article also appears to have a promotional tone towards the proposed deep learning technique. While it is understandable that researchers may want to highlight their novel approach, it is important to present a balanced view and acknowledge the limitations and potential risks associated with the method. This lack of critical discussion raises concerns about the objectivity and reliability of the findings.
In conclusion, this article presents an interesting approach to clustering histopathology images of cholangiocarcinoma using deep learning techniques. However, it lacks critical analysis, evidence to support its claims, consideration of confounding factors, and discussion of potential limitations and risks. Further research and validation studies are needed to determine the clinical utility and generalizability of these clustering methods in predicting patient outcomes.