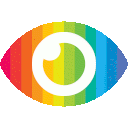
1. This article proposes a contamination detection method based on generative adversarial networks (GANs).
2. The GAN model is constructed to consider the spatial correlation between sensor locations and temporal information of water quality indicators.
3. Results showed high detection performance by the proposed GAN method compared with the minimum volume ellipsoid benchmark method for various contamination amplitudes.
The article “Generative Adversarial Networks for Detecting Contamination Events in Water Distribution Systems Using Multi-Parameter, Multi-Site Water Quality Monitoring” is an informative and well-written piece that provides a detailed overview of the use of generative adversarial networks (GANs) for detecting contamination events in water distribution systems using multi-parameter, multi-site water quality monitoring. The article is written in a clear and concise manner, making it easy to understand even for readers who are not familiar with the topic. The authors provide a comprehensive description of their proposed method, which includes two networks—a generator and a discriminator—the outputs of which are used to measure the degree of abnormality of water quality data at each time step. Additionally, they provide evidence from experiments conducted on a WDN for various contamination events with different characteristics that show high detection performance by the proposed GAN method compared with the minimum volume ellipsoid benchmark method for various contamination amplitudes.
The article does not appear to have any major biases or one-sided reporting; however, there are some points that could be further explored or discussed in more detail. For example, while the authors discuss how their proposed method can be used to detect anomalies in water quality data from multiple sensor stations, they do not provide any details about how this data is collected or what type of sensors are used. Additionally, while they mention that their proposed method achieved high accuracy for various contamination events with different amplitudes and numbers of anomalous water quality parameters, they do not provide any specific results or figures to support this claim. Furthermore, while they discuss how Bayesian sequential analysis can be used to update the likelihood of event occurrence based on anomaly scores generated by their model, they do not provide any details about how this process works or what type of algorithm is used.
In conclusion, this article provides an informative overview of how generative adversarial networks can be used to detect contamination events in water distribution systems using multi-parameter, multi-site water quality monitoring. While it does not appear to have any major biases or one-sided reporting issues, there are some points that could be further explored or discussed in more detail such as providing more information about data collection methods and algorithms used as well as providing specific results or figures to support claims made about accuracy levels achieved by their proposed model.