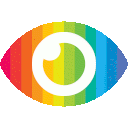
1. Multimodal deep learning methods show promise in predicting overall survival and other prognostic factors in glioma patients.
2. The integration of MRI imaging with clinical, genomic, and histologic data improves the accuracy of prognostication models.
3. However, there are limitations in data availability and reporting transparency that need to be addressed for the full potential of multimodal deep learning in glioma prognosis to be realized.
The article titled "Multimodal Deep Learning-Based Prognostication in Glioma Patients: A Systematic Review" provides a comprehensive overview of the current state of deep learning (DL) methods for predicting prognosis in glioma patients. The authors conducted a systematic review of the literature and identified 16 studies that used DL models to predict overall survival, genotype characteristics, and response to immunotherapy in glioma patients.
Overall, the article provides valuable insights into the potential of multimodal DL models for improving prognostication in glioma patients. However, there are several limitations and biases that should be considered when interpreting the findings.
Firstly, the article acknowledges that there is a risk of bias in the included studies, particularly due to inconsistent methodological reporting. This raises concerns about the reliability and reproducibility of the results. Without clear reporting of methods and data sources, it is difficult to assess the validity of the findings.
Additionally, while the article highlights the potential benefits of using multimodal data in DL models, it does not adequately address the challenges and limitations associated with integrating different data modalities. For example, there may be issues with data quality, compatibility between different data types, and selection bias in choosing which modalities to include. These factors can significantly impact the performance and generalizability of multimodal DL models.
Furthermore, the article does not thoroughly explore potential risks or limitations associated with using DL models in clinical practice. DL models are known for their complexity and lack of interpretability, which can make it challenging for clinicians to trust and understand their predictions. Additionally, DL models are highly dependent on training data, which may vary across different clinical settings. These factors should be carefully considered before implementing DL models for prognostication in glioma patients.
Another limitation is that the article primarily focuses on studies that predict overall survival as an outcome measure. While overall survival is an important endpoint in cancer prognosis, it may not capture the full complexity of glioma patients' outcomes. Other factors such as quality of life, functional status, and treatment response should also be considered in prognostication models.
Lastly, the article does not provide a balanced discussion of the potential limitations and challenges associated with DL models. It primarily highlights the benefits and improvements achieved by multimodal DL models without adequately addressing potential drawbacks or alternative approaches. A more comprehensive analysis that considers both the advantages and disadvantages of DL models would provide a more nuanced understanding of their utility in glioma prognostication.
In conclusion, while the article provides valuable insights into the use of multimodal DL models for predicting prognosis in glioma patients, it has several limitations and biases that should be considered. Future research should address these limitations and provide a more balanced assessment of the potential benefits and challenges associated with using DL models in clinical practice.