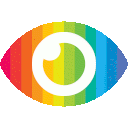
1. Social media check-in data can be used to analyze urban structure and socioeconomic performance.
2. A novel framework was proposed to characterize urban streets with land-use connectivity indices measured by a place-function signature.
3. The introduced metrics were found to be more effective than traditional methods for describing housing price patterns.
The article "Urban function connectivity: Characterisation of functional urban streets with social media check-in data" presents a novel framework for characterizing urban streets using social media check-in data. The authors propose a new type of place-function signature to measure land-use connectivity indices and introduce a composite index that packages three principal dimensions of urban function network. The study aims to advance our knowledge of network accessibility and portray urban structure and related socioeconomic performance more explicitly.
The article provides valuable insights into the potential use of social media data in urban studies. However, there are some potential biases and limitations in the study that need to be considered. Firstly, the study is based on location-based social media check-in data, which may not represent the entire population's preferences or activities. The sample size may also be limited, leading to sampling problems and context-related uncertainty.
Secondly, the study focuses on street-based function regions and does not consider other factors such as transportation modes or environmental factors that may affect accessibility. This limitation may lead to an incomplete understanding of network accessibility and its impact on socioeconomic performance.
Thirdly, the study only considers one city (Tianjin, China), which limits its generalizability to other cities or regions with different characteristics. The results may not be applicable to other contexts without further validation.
Fourthly, while the article claims that their metrics are favorably comparable than traditional methods for describing housing price patterns, it does not provide sufficient evidence or comparison with other studies' findings.
Finally, the article does not explore counterarguments or potential risks associated with using social media data in urban studies. For example, privacy concerns or biases in social media usage may affect the validity of the results.
In conclusion, while this article provides valuable insights into using social media data for characterizing urban streets' functionality and network accessibility, it is essential to consider its limitations and potential biases carefully. Further research is needed to validate these findings in different contexts and explore potential risks and limitations associated with using social media data in urban studies.